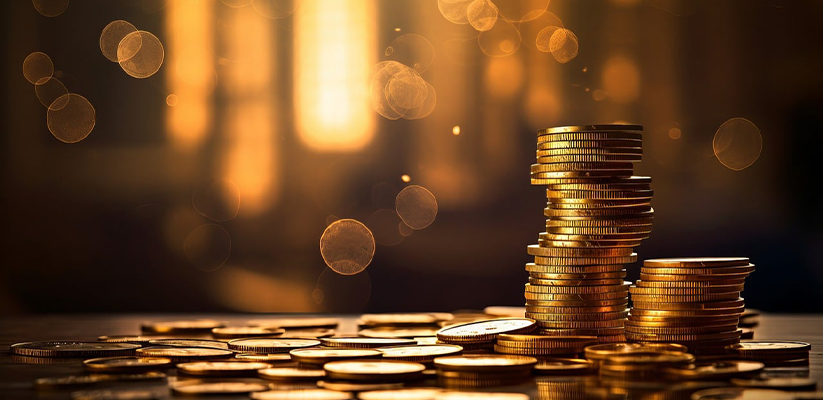
In the realm of retail banking, data-driven insights reveal what customers do, but they often fail to capture why they make certain decisions. This “why” encompasses the psychological drivers—like loss aversion, herd mentality, and emotional biases—that significantly shape financial behaviors. Despite having vast amounts of customer data, many banks struggle with engagement and trust because traditional data approaches overlook these underlying motivations.
To bridge this gap, banks can prioritize user-centric design informed by behavioral insights, creating experiences that resonate emotionally as well as functionally. By blending data analytics with behavioral psychology, banks can foster trust, transparency, and ease of navigation, improving customer satisfaction and loyalty. This approach to personalization has the potential to transform customer relationships, setting a new standard for customer-centric innovation in banking.
To drive innovation and customer engagement, retail banks must integrate behavioral science, user-centric design, and real-time feedback. Key actions for banking CIOs include building teams with behavioral science skills, implementing a Behavioral Insights Dashboard for personalized experiences, and designing user-friendly data frameworks to reduce decision fatigue. Establishing feedback loops and embedding emotional intelligence in AI ensures responsive, personalized interactions. Segmenting data by customer lifecycle, using gamification to encourage positive habits, prioritizing data privacy, and adopting a test-and-learn approach are also crucial for continuous improvement and trust-building.
Collectively, these strategies would create a well-rounded approach to engaging customers on practical and emotional fronts, positioning banks as agile, customer-centric competitors.
“Consumers often make financial decisions based on behavioral biases rather than pure rationality. Understanding the psychological factors as to why decisions are made, such as loss aversion or herd mentality, can enhance the effectiveness of teams in designing customer-centric solutions,” says Gartner, in “Maximize Competitiveness in Banking with Behavioral and Data Science.”
Clearly, banking CIOs must go beyond data analytics by integrating behavioral science into their strategies. This would enable banks to develop more personalized, effective customer experiences. It’s time for banking leaders to apply these principles to stay competitive and deliver superior customer value.
How Does It Work?
Behavioral science examines how people make decisions, often diverging from purely rational choices, by identifying underlying psychological drivers. It works how it works by identifying biases, motivations, and emotional triggers. Using models like prospect theory, choice architecture, and experimentation, it guides customers toward positive financial behaviors through subtle nudges and tailored responses. This approach creates trust and enhances customer experience by aligning services with human tendencies and ethical practices.
To apply this approach, banks need access to diverse data sources that capture customer behaviors, preferences, and motivations, which include:
- Transaction Data: Reveals spending, savings, and investment patterns, providing insights into financial habits like risk tolerance.
- Customer Demographics: Offers age, income, and lifestyle details for tailored services and segmentation.
- Customer Interaction Data: Tracks engagement across various channels to improve customer service.
- Digital Behavior Data: Monitors online interactions to optimize interfaces and feature usage.
- Social Media Data: Gauges customer sentiment and brand perception from reviews and social platforms.
- Credit and Loan Data: Assesses debt management and financial discipline for personalized advice.
- Marketing Data: Analyzes responses to promotions to understand purchasing influences and loyalty.
- Customer Feedback: Collects satisfaction and expectations to enhance loyalty and services.
- ATM and POS Data: Tracks cash vs. digital preferences and geographic behaviors.
- Real-Time Event Data: Monitors responses to market changes, crucial for decision-making under stress.
- Third-Party Data: Provides insights from external partners for broader behavioral analysis.
- Geolocation Data: Identifies regional trends to inform targeted offerings.
- Customer Support Data: Highlights common issues and service expectations for improved experience.
The Role of Logical Data Management
Capturing this information, to better understand customer motivations and behaviors, is not easy, but a logical data management approach is a strong starting point. Logical data management solutions use data virtualization to manage data without having to replicate it into a single repository. Logical data management solutions provide banks with seamless, real-time access to diverse data sources, supporting adaptive feedback, accurate customer segmentation, and streamlined data governance for privacy and compliance. This encourages rapid testing, fosters innovation, and powers AI models with high-quality data, enabling banks to deliver responsive, customer-centric solutions.
With a logical data management platform, banks can gain a holistic view of customer behavior by integrating both structured and unstructured data sources. This approach enables real-time insights for personalized interactions, seamlessly bridges legacy and cloud systems without costly migrations, and simplifies data integration to save time and resources. Logical data management platforms also strengthen governance and security, facilitating compliance and building customer trust. By empowering real-time AI model adaptation with reliable data, logical data management platforms enable banks to effectively apply behavioral insights, positioning them as agile, customer-focused, and trustworthy institutions.
Logical data management platforms enable two key innovations:
- Data fabric, which forms a real-time data access layer across an organization’s diverse data sources.
- Data products, which are data-centric applications tailored to meet the needs of particular users or user groups.
Logical Data Management and Behavioral Science for Banking Customers
With a logical data management platform, organizations can build a wide variety of data products that might incorporate behavioral science data for banking customers. Let’s look at a few examples:
Personalized Financial Health Dashboards could provide customers with tailored overviews of their financial habits, including spending, saving, and credit usage. By leveraging behavioral insights, such as loss aversion or impulsive spending patterns, such dashboards would offer advice to improve financial health, nudging customers towards better habits like saving more or reducing debt. For example, the dashboard might highlight potential savings from reducing unnecessary expenses, reinforcing future rewards like a vacation or a major purchase.
Customer Journey-Based Credit Scoring Models could enhance traditional credit scores by incorporating behavioral data like spending patterns and risk tolerance. These models would provide a more accurate picture of creditworthiness by analyzing habits such as late payments or impulsive spending. For example, a young customer with a short credit history but responsible financial behavior might still receive a favorable score, reflecting their low risk.
Behaviorally-Driven Investment Recommendation Engines could offer personalized investment advice by combining financial data with behavioral insights into risk tolerance, financial goals, and emotional reactions to market changes. Such engines would align investment strategies with a customer’s psychological profile, helping to reduce anxiety and build trust. For example, for risk-averse customers, they might suggest a diversified portfolio with more stable investments, such as bonds.
Real-Time Spending Alerts with Behavioral Triggers could instantly notify customers when their spending deviates from usual patterns, encouraging smarter financial choices. By leveraging behavioral insights, such as impulse buying or emotional spending, such alerts would help customers stay on track. A system might, for example, remind a customer to stay within budget during holiday shopping, if it detects overspending tendencies.
Savings Gamification Tools could leverage behavioral science to create engaging, game-like experiences that help customers reach their savings goals. By offering rewards, challenges, and progress tracking, such tools would motivate users through competition and incentives. Customers would earn badges or small rewards for hitting savings milestones, with regular nudges to keep them on track, making the process of saving more interactive and enjoyable.
Personalized Loan Offers with Behaviorally-Driven Terms could customize loan conditions based on both financial standing and behavioral factors like spending habits, income stability, and financial goals. Behavioral data would help predict repayment reliability, enabling banks to offer better terms, such as lower interest rates, to financially responsible customers. For example, a customer with consistent credit card payments might receive a better loan rate than someone with similar income but less reliable financial behavior.
AI-Driven Chatbots with Emotional Intelligence could provide personalized customer support by analyzing behavioral data to understand the emotional state of the user. Such chatbots might adapt their tone and suggestions to more effectively address customer needs, such as offering calm advice during moments of stress or uncertainty. If a customer is anxious about finances, the chatbot might suggest helpful steps like setting up a payment plan or managing bills. in a supportive manner.
Fraud Detection Systems with Behavioral Profiling could monitor customer behavior to detect fraud by identifying unusual patterns. Such systems might use behavioral data to flag activities like sudden large purchases or transactions from unfamiliar locations, which deviate from a customer’s typical behavior. If a customer known for making small, local purchases suddenly spends a large amount abroad, the system might trigger a fraud alert.
Such data products would enhance customer experiences in banking by using behavioral science to offer personalized, real-time solutions that align with individual habits, preferences, and financial goals.
Rewards for Good Behavioral Science
Integrating behavioral science into customer engagement strategies is no longer just an option for retail banks—it’s essential. By leveraging insights from customer behavior, emotional intelligence, and user-centric design, banks can deliver hyper-personalized experiences that build trust, encourage loyalty, and drive meaningful innovation. From personalized financial dashboards to AI-powered chatbots, the opportunities to create deeper, more impactful customer relationships are vast. Now is the time for banking leaders to invest in behavioral science tools, prioritize real-time feedback, and continually evolve their strategies, knowing that a logical data management platform can ensure that access to all relevant data is governed, immediate, and secure.
- Holding Back the Data Tide: Why Logical Data Management is No Longer Optional - April 16, 2025
- AI Can’t Save Lives If Healthcare Data Stays Broken - April 3, 2025
- Developing a Practical, Value-Driven GenAI Strategy for Insurance - March 20, 2025