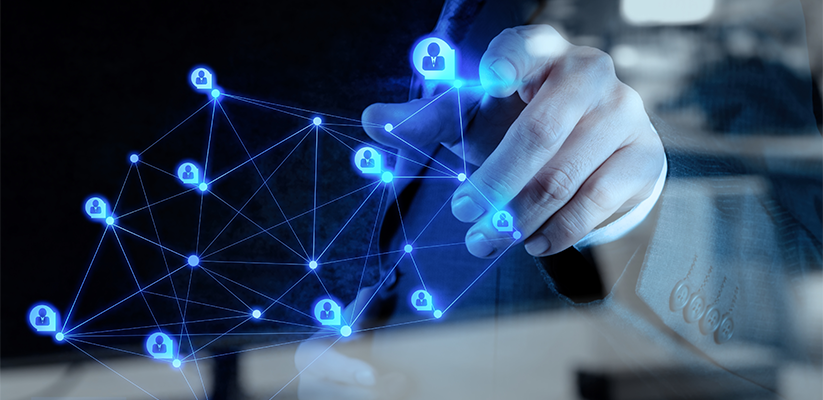
One of the biggest challenges for organizations is to integrate data from various sources. Despite modern advancements such as big data technologies and cloud, data often ends up in organized silos, but this means that cloud data is separated from on-premises data, modern data is separated from legacy data, etc. Bringing all the disparate data together takes time and resources. Often, data needs to be moved to a physical repository to be warehoused and managed. Alternatively, the data can be continuously integrated, but this is an expensive process. This is where data virtualization comes in, a unique data integration technology that integrates data without moving it.
Data virtualization provides data integration in an agile manner, providing a unified view of disparate data sources. Data virtualization introduces a logical layer between data providers and users. This layer can retrieve data on-demand, transforming and combining data virtually to deliver necessary data to users. Data virtualization is a technology that does not move data between various sources but is still able to logically integrate data to provide a unified view for effective user consumption.
Understanding Traditional BI vs. Agile BI
Before I further discuss data virtualization, I want to take a step back and briefly explain the difference between agile business intelligence (BI) and traditional BI, and the impact of agile BI on evolving business needs.
Traditional BI relies heavily on extract, transform, and load (ETL) processes, in which data is sourced and copied into a data warehouse. Over time, however, this process of moving and preparing data for consumption becomes slow and costly for organizations. In most cases, the data needs to be physicalized, copied, or replicated into another location, to suit each specific use case.
This method is still a valid approach in some cases where moving and modeling data through different layers or data architectures is needed. However, using a logical approach, rather than a physical approach, can be both more cost-effective and faster. In contrast to traditional BI, agile BI is more flexible and dynamic, capable of quickly adapting to changing business needs.
Creating an Agile BI Infrastructure with Data Virtualization
Data virtualization creates a logical data layer between the source data and the consuming applications, BI tools included. This removes the complexities of where the data comes from and how it is combined or aggregated — all of this is maintained in a single place. The Denodo Platform, powered by data virtualization, provides an agile, high performance logical data integration platform across the broadest range of enterprise data assets.
Anyone working in BI knows that as soon as data is delivered, there is often a requirement for more data, or for something different. So, the agility of data virtualization is needed to address any new business needs with data, quickly and easily.
Effective Agile BI Initiatives with Data Virtualization
An agile approach to data integration, enabled by data virtualization, helps us to not repeat any processes unnecessarily. For example, when building a report using a BI tool, we first connect to each of the sources, build the logic for combining the source tables, build the logic to prepare the data, and then build more logic to display or visualize the data. Each BI user is doing the same things over and over again, whereas the business logic should ideally be defined and maintained in a single place as much as possible, to be re-purposed or re-used.
To avoid this repetition, data virtualization uses a logical data integration approach that accelerates data delivery, enabling businesses to access data faster and therefore react to changes more quickly and easily.
For more insights on how to create an Agile BI infrastructure with Data Virtualization, check out our discussion with Katrina Briedis, Senior Product Marketing Manager (APAC) at Denodo for more information on All Things Data!
- Embracing Data Mesh: A Modern Approach to Data Management - September 21, 2023
- Monolithic vs. Logical Architecture: Which for the Win? - February 16, 2023
- Modern Data Ecosystems that Drive Business Value - January 6, 2023