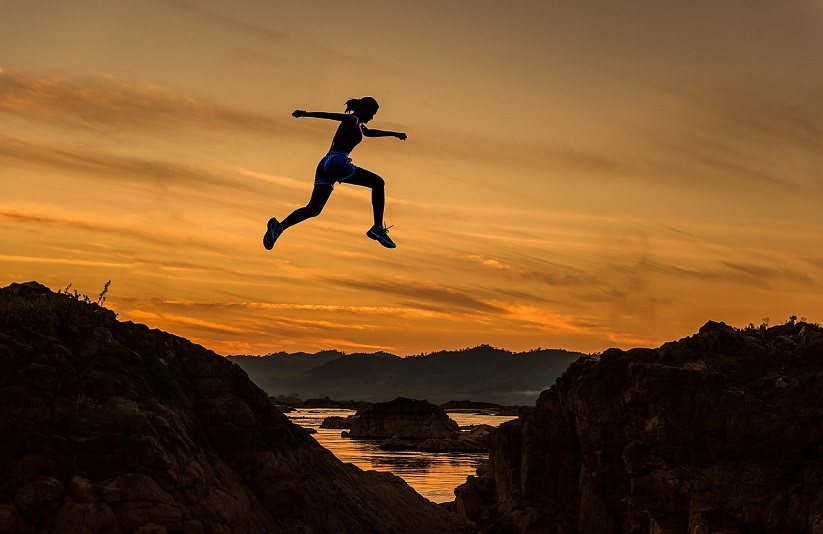
How many users, inside and outside your organization, access your organization’s data? Dozens? More likely you have a hundred or more, each with his or her own structure and content requirements. Moreover, users probably have different rights, in terms of what they are allowed to see. Over the years, many organizations have therefore witnessed the formation of “data delivery mills,” in various shapes and sizes. How does one create order and reliability in this world of chaotic data streams? Quite easily: with a data virtualization platform.
In commercial organizations, data sets are submitted to clients and suppliers, and each party receives data at regular intervals, in tailor-made, usable format. The data files are analyzed or used as a source for the in-house management information system. Some organizations are even obliged to submit data to government bodies, audit bodies, industry partners, or umbrella organizations due to their market position or because of legislative requirements. In such cases, the data is often highly confidential.
Most organizations use a central data platform, such as a data warehouse, when delivering data sets. In most cases, the platform leverages a “traditional” data integration architecture, in which source data is physically copied, transformed, and integrated via an ETL tool. The data is drawn from ERP systems, CRM applications, cloud applications, flat files, and other sources. The central, integrated data platform (or data warehouse) then becomes the source for all data sets. The same ETL tool also produces the physical tables and files, and sends them out, in line with the relevant agreements. In some cases, steps in this process have been rendered “logical” with database views.
Does this describe your situation? If so, you are undoubtedly facing at least one of these four challenges:
1. Chaos
You probably began your data delivery initiatives on a foundation of sound ideas, plans, and intentions. However, somewhere along the line you may have come to the realization that you no longer have control, and that the situation has become chaotic. Imagine that you deliver an information product to your clients, in the form of files, with all the data on their client portfolios. You started out with one or two clients who wanted to receive data sets once or twice a month. To meet these demands, you established an environment that enabled you to meet all the needs and requirements of your clients, and you took the growth of this environment into account. But a few years down the road… your organization has grown, and you have become increasingly professional. Various consequences:
- Scale: The number of clients you deliver to has increased dramatically.
- Customization: The demands of a few of your clients deviate in terms of the structure and content of the files.
- Version management: Some clients cannot apply changes to their environment with the same frequency as your changes to the delivery of data.
- Auditability: Once files have been sent out, the law requires that they remain available for a specific period of time.
- Frequency: It is no longer acceptable to send out an updated data set once a month. Your clients want data at least weekly or daily, and some even want real-time data.
These consequences have a major influence on the complexity of your environment. The situation in which exceptions are no longer needed for specific clients (and you have the ability to serve all users through one approach) does not exist. After all, the customer is king. So, the data streams grow into a collection of client-specific exceptions and workarounds. Nobody is aware of the exact differences between clients, and a great deal of the knowledge is kept in the heads of a few employees. No one no longer knows how many accurate files are being sent out, or even if these files are still being used. Also, adding to (or adjusting) your data delivery to a client becomes a project in itself.
2. Labor-intensiveness
For a traditional data platform, it takes a relatively long time to implement a new data delivery or to adjust an existing data delivery. After a functional and technical analysis, a development process must be started so that the new ETL process can be developed, tested, accepted, and commissioned for the specific delivery. This does not yet even take into account functional acceptance, rework based on test results, documentation, or follow-up care. All in all, it takes a great deal of work to achieve one data delivery.
3. Intensive Management
The growing number of clients and data deliveries also has significant consequences for the scope and complexity of the management of all data streams. Moreover, the volume on your data platform increases dramatically over time. More and more clients want to receive increasing volumes of data. All of the supplied data is replicated and stored in one way or another. In other words, data volumes don’t grow gradually, but exponentially. More data and increased data deliveries automatically lead to more management, until all of the leeway is gone. The environment becomes way too expensive, and problems can no longer be solved fast enough, resulting in incomplete data sets being delivered, late delivery, and even incorrect data.
4. Limited Governance
When you work with confidential data, the rules and regulations seem to be ever increasing. There are substantial risks and fines associated with the incorrect use of data and data breaches, and it is a constant challenge to update your data platform to accommodate this. Today, legislative requirements are taken into account at the design phase. The main question used to be “what does the client want?” Now, the questions are “what does the client want, is the client allowed to have it, and how safe is it?”
Laws and regulations pose two challenges. When compiling your data deliveries, you must take great care with what the recipient is entitled to see. There could also be multiple recipients! In addition, your environment must be auditable. You must record the origin of each data element and the way in which the information was generated. You must be able to demonstrate that the recipients of the information are entitled to view the (compiled) information. Even if your information is not privacy-sensitive, data governance is still a very relevant subject. It is disastrous if a user is able to view information that is not intended for him or her, or makes decisions based on incorrect or incomplete data.
A data virtualization platform solves these four problems and helps you create order in the chaos and data deliveries. Four problems solved in one go!
Data virtualization unlocks and integrates data from various sources, in a logical way, and makes it available to receiving systems, without replicating any data. This technology produces the same end result as that achieved with traditional ETL processes, in which data is physically replicated. Data virtualization helps you to get your data deliveries done more efficiently and gives you more flexibility to implement changes with less management effort. Moreover, you can easily provide insight into how data sets were created, who has access to what data, and what information is being requested.
1. Not Chaos, But an Overview
With a data virtualization platform, you can basically perform all of your data deliveries without having to replicate any data. If you have a central data platform that provides an auditable history with traceability, you can connect it to the data virtualization platform as a data source, which will replace all instances of physical ETL tools generating data sets, so complexity is significantly reduced. You can unlock new data sources via the traditional data platform, but you can also connect them directly to the data virtualization layer. You then use the layer when publishing data sets to users.
With the authorization possibilities of a data virtualization platform, you maintain full control over who has access to what data. Since the virtual environment covers your existing data warehouse, you can simply continue to deliver the same data sets, but now they are based on one set of virtual tables. Filters are subsequently linked to users and user groups, so that each user only sees the data intended for them. If users have deviating needs or requirements, one can usually accommodate them quite easily, by merely implementing authorization rules. If that is not sufficient, you can create a new virtual table, in the wink of an eye, including transformations and links.
2. Rapid Development
It takes less time to develop new data deliveries with a data virtualization platform, compared with a traditional (physical) environment. After all, all you have to do is design and prototype. The content and structure of the table are determined in the design phase. You then define the required table via joins, transformations, and filters, in the virtual layer. Since the table is virtual, the result is viewed immediately and you can coordinate with your users right away, to see if the information meets their needs and requirements.
3. Limited Management
With data virtualization, it is much easier to manage the data deliveries. Data is not replicated and the data volume grows at a minimal rate, so the costs for technical management of your data platforms are considerably lower than for physical replication. Since you use the virtual tables and smart authorization, the logic in the platform is much more transparent. This dramatically reduces the number of data sets that just “lie about” in your organization.
4. Governance Is Supported
A data virtualization platform offers extensive capabilities for tracking logging, impact, and lineage. In combination with the authorization capabilities, these also enable you to see exactly where information comes from and how it was generated. Moreover, the platform provides insight into who accessed what information and who has what type of authorization. The platform therefore gives you all the means with which to establish your data deliveries in a transparent yet auditable manner, in combination with your existing data warehouse.
Some might think that a data virtualization solution would lead to performance-related problems. However, the new generation of data virtualization platforms provide a powerful, smart “virtual motor” filled with intelligence, enabling large-scale virtualization without any significant impact on performance.
In this respect, data virtualization is the same as server virtualization. It is only a matter of time before data virtualization technology becomes a standard part of each organization’s information landscape.
This post was submitted by Mattijs Voet, Senior Consultant, Kadenza
- How Much Time Could Your Company Save If You Said Goodbye to Data Migration? - January 30, 2019
- Get Ready for the General Data Protection Regulation (GDPR), with Data Virtualization - May 24, 2018
- Data Virtualization is a Revenue Generator - September 20, 2017