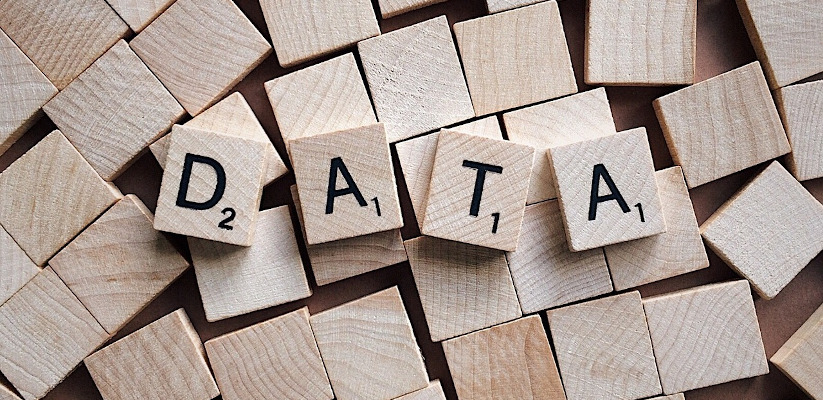
By Barry Devlin
“I want it all, and I want it now,” sang Freddie Mercury in “I Want It All.”
Today’s businesspeople, it turns out, feel just the same way. The pace of business has ramped up dramatically over the last few years. Customers expect next-day delivery or better. Supply chains are increasingly real-time. And executives need to know what’s happening now. Or even what is going to happen next.
Decision-making needs are becoming more urgent, demanding more flexibility than ever before in many areas of today’s business. This is even true to some extent in regulatory reporting, not typically the department most in need of real-time decision making. Such urgency and flexibility demands fresher data than most traditional business intelligence (BI) systems can deliver. Indeed, data warehousing has long recognized a fundamental trade-off between data timeliness and consistency: it takes time to reconcile differences in data coming from different sources. Consider also that for some older systems—particularly in the financial services industry—end-of-day batch reconciliation processing of the day’s transactions is still common.
Nonetheless, fresher data sources are becoming more widely available to many business decision makers. In more traditional businesses, IT stakeholders are beginning to recognize that they need to provide agile access—albeit constrained by performance and security considerations—to operational systems for decision-making support. Data streaming in from clickstreams, social media, and Internet of Things (IoT) devices has grown manyfold since the early years of the new millennium, and such data rapidly loses value as it ages. Decisions based on it must be made with urgency or not made at all. And businesspeople need the agility to change their minds at will.
The bottom line is that flexible decisions need fresher data and agile delivery; and fresher data demands flexible decisions and agile delivery. As fresher data becomes available, decision makers and data scientists will find ways to access and use it by any means possible, often to the detriment of good data governance. The best IT teams are challenged by a combination of business needs and data challenges, and they are taxed both organizationally and technically.
What’s a Good IT Team to Do?
In a modern digital business, a key responsibility of IT is to balance the agile delivery of more, fresher data with the need to govern, and manage responsibly, all the data used by the business. This means making data from both traditional and non-traditional sources available to the business in a controlled manner. It does not mean delivering non-traditional data to the business in the same way as traditional data. In fact, it is likely impossible to deliver some new forms of data through the traditional data warehouse architecture, because that would require all of it to pass through the bottleneck of an enterprise data warehouse (EDW), an architecture that is not renowned for agility!
In order to offer fresh data to the business in the volumes business stakeholders require, the traditional decision support approach of making copies for specific purposes simply cannot work. For such data, IT must move away from extract, transform and load (ETL) thinking to an “access in place” model. This immediately implies that there will exist multiple, disparate data stores that businesspeople will need to visit to find the fresh data they need. In addition, other data will continue to be accessed in existing data warehouses and marts. Not only will data need to be accessed across these diverse environments, but it will need in some cases to be joined across them.
Data Virtualization to the Rescue
Data virtualization addresses these various demands.
First, its premise is the exact opposite of that of data warehousing; data virtualization provides direct access to all data sources—externally sourced, operational, data warehouse originated, and more—for businesspeople and their apps, rather than requiring up-front consolidation to a single store. Minimal planning and IT project work is required before new sources can be accessed.
Second, by offering such direct access and immediate user acceptance testing of the outcome, data virtualization is inherently more agile than ETL / EDW approaches that require bigger and longer projects.
Third, data virtualization allows immediate access to fresh data as soon as it arrives in the enterprise, avoiding the delays inherent in traditional approaches. Subject to the understanding that such data may be incomplete, inconsistent, or in need of cleansing, businesspeople can use it for instant analysis and decision making.
Fourth, it offers the possibility to join data across diverse sources via an initial modelling phase in which IT can apply governance and data management principles. After that, businesspeople can—if they so wish or need—create their own apps or modify existing ones, accessing and joining data as changing business needs demand.
You Can Have It All
Taken together, these four benefits of data virtualization offer support for flexible decision making based on both fresh and pre-consolidated data, delivered with agility, all with proper data governance, where appropriate.
Freddie Mercury, I suspect, would be delighted!
- The Data Warehouse is Dead, Long Live the Data Warehouse, Part II - November 24, 2022
- The Data Warehouse is Dead, Long Live the Data Warehouse, Part I - October 18, 2022
- Weaving Architectural Patterns III – Data Mesh - December 16, 2021