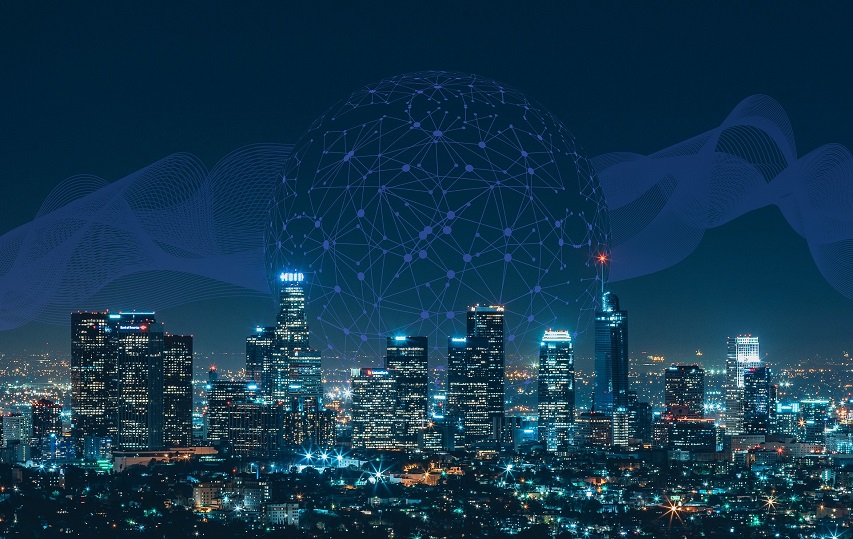
In the Bronze age, recycling became large-scale, opening the doors to cross-border trade and collaboration across nations. Unwanted bronze implements were melted down and poured into a mold to make new implements, and thus the manufacturing technology of the bronze age was transformative rather than reductive. It supported what we now call a circular economy, though today’s circular economy is AI-based. For example, predictive maintenance enables manufacturers to offer customers the option to not only repair their products as needed, but also to resell them, refurbish them, or recycle them.
The Critical Collaboration Factor
Predictive maintenance, however, requires the means to collaborate and share data in a secure manner to protect the privacy of individuals who are reluctant to exchange equipment failure data with manufacturers or third parties. Also, the data would need to be combined across spare part databases, recycling prices, and the product reuse marketplace. Many industries are highly regulated, and new privacy and security laws like the GDPR have put additional strains on this challenge.
Without being able to easily share training data, such as policies, cross-sectoral regulations, and cross-border regulations, organizations would find it challenging to build insightful machine learning models to drive more efficient product re-circulation, reuse, and recycling. Privacy-preserving, decentralized, collaborative machine learning techniques are simply not possible using traditional data processing systems that perform data collection, data cleansing, data integration, and model transfer without a standard framework.
Federated Learning Meets Data Virtualization
Federated learning, originated by Google in 2017, makes it possible to build collaborative machine learning models without direct access to training data. This preserves privacy, and creates lighter workloads without requiring that the data be moved from its original location.
Data virtualization is a data integration method that can integrate data sources also without requiring data movement. Can federated learning and data virtualization work in harmony? And can federated learning be improved and optimized with the help of edge computing and data virtualization?
The Necessary Framework
Note that it will not be easy to increase aggregated learning accuracy in the cloud without extensive back-and-forth communications to and from the cloud server, which would cause propagation latency and intensive data transmissions, degrading model performance and saturating the backbone of the network. Academics and industry stakeholders are continuously improving the algorithmic efficiency of federated learning, but these improvements will not suffice until we build the right hybrid edge and data virtualization architecture in support of federated learning.
In the original federated learning framework, new learnings and discoveries are aggregated and sent from mobile phones or IoT devices to the cloud, but with a proper multi-access edge computing (MEC) platform, low-cost learning can be accomplished locally at the edge before performing the global aggregation on the cloud level.
The framework illustrated above will surely surmount many enterprise challenges in achieving data privacy, but in order to become successful in digital transformation as a whole, organizations will need to have “Data Readiness,” which is trust in the organization’s data quality combined with enterprise-wide, privacy-preserving data access, so that data is always ready for anyone inside or outside of the enterprise to use.
Data virtualization can be deployed at any intermediary layer between the edge and data center or the cloud, including organization and region based layers, to establish seamless communication and data integration among federated learning instances, the edge, and cloud servers. It works by abstracting data sources and creating a unified view of all the information, augmented by a data catalog that can be accessed for customer facing apps, specifying what parts of the data can be seen, and what types of data is allowed to move cross-border or cross-sector. As such, the data virtualization layer can act as the holistic enterprise-wide privacy-preserving layer (for data federation). On the other hand, federated learning can ensure privacy for all sandbox and customer production AI and machine learning applications, acting as the local privacy-preserving layer for AI apps (federation of the algorithmic learning).
A New Age of Transformational Technologies
With all of the maturity and consolidation happening in the data management and analytics market today, enterprises have access to a wide variety of new analytics tools. However, fostering a collaborative data environment is the single most critical change that could make their digital transformation efforts a success, enabling a circular economy that leverages the transformative aspects of the bronze age.
- If Trust is the Main Ingredient of Leadership, Is Trust the Main Ingredient of Successful AI? - November 18, 2020
- The Main Pillars of Future Polymer Factories, the Next Future Material for AI - November 15, 2019
- From the Bronze Age to Today’s AI-based Circular Economy, and How Data Virtualization and Federated Learning Make It Possible - August 13, 2019