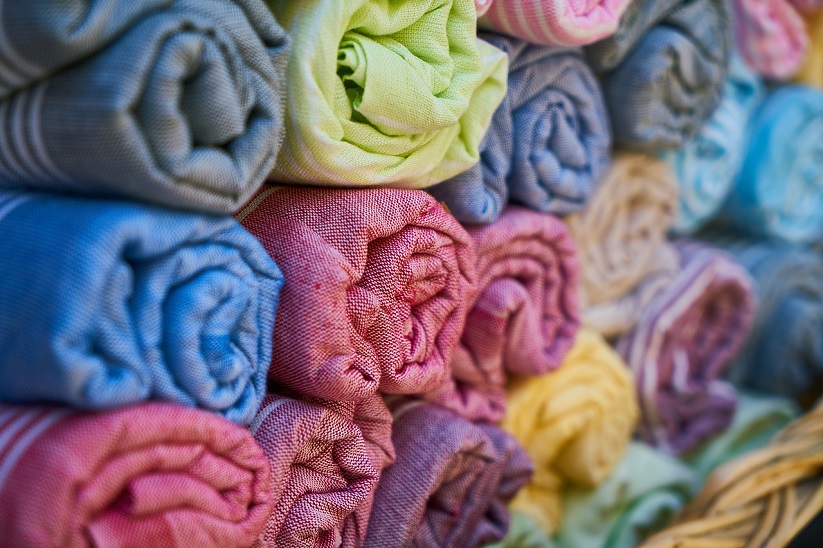
Challenges to Enterprise-wide Adoption of Big Data Technologies
Lack of comprehensive strategic planning is proving to be the greatest impediment to enterprise-wide adoption of big data technologies. While organizations are quick to invest in new technologies like Hadoop cluster and NoSQL database for collecting a wide variety of disparate data that is now available from a myriad of new data sources, they are not concurrently addressing downstream integration and governance challenges that must be overcome to drive corporate-wide big data adoption, expansion to multiple use cases, and maturity.
Some key challenges faced by the champions of big data adoption in their organizations are highlighted below.
- Lack of flexible and coherent architecture – In conjunction with supporting legacy data sources, modern data architectures must accommodate new, disparate data sources that provide diverse types of data. Whereas ingestion of disparate data into big data stores like Hadoop is relatively straight-forward, gaining access to this multi-structured data in a flexible manner is more complicated.
- Skills scarcity – Organizations are finding it difficult to match existing skill sets with the demands of new big data technologies.
- Metadata – Availability of metadata and its integration with other metadata repositories is inadequate.
- Data governance – Whereas traditional data sources are well-governed, big data sources are characterized by a lack of data stewardship, policies, and security.
- Sustained funding – Establishing early wins with proof-of-concept (POC) big data analytics projects, especially those that clearly demonstrate tangible business value, can be crucial to securing IT and Business buy-in and sustained future funding.
Organizations must tackle these and other hurdles up-front as part of a comprehensive strategy supporting adoption of big data technologies.
Big Data Fabric Drives Enterprise Adoption
Big data fabric offers a comprehensive approach to overcoming the challenges hampering adoption of big data technologies.
What is Big Data Fabric? A big data fabric enables global access to all data assets of an enterprise, leveraging storage and processing power from multiple heterogeneous nodes.
“Bringing together disparate big data sources automatically, intelligently, and securely, and processing them in a big data platform technology, such as Hadoop and Apache Spark, to deliver a unified, trusted, and comprehensive view of customer and business data.” Forrester (Big Data Fabric Drives Innovation And Growth, March 8, 2016)
Data Virtualization Epitomizes Big Data Fabric
Data virtualization is the ideal choice for big data fabric because it provides a comprehensive set of capabilities within a single platform to facilitate and promote big data discoveries in a seamless manner.
In response to the challenges underlined below, data virtualization:
- Coherent architecture – offers a common access point for all big data plus traditional data sources by exploiting the processing power of underlying big data technologies such as Apache Hadoop and Apache Spark.
- Skills scarcity – hides the complexity of, and facilitates access to big data, thus bridges the skill set gap by minimizing the need to learn special languages or decipher a variety of data formats.
- Metadata – furnishes enriched metadata, including data lineage, which facilitates understanding and discovery of the underlying data assets.
- Data governance – enables governed data discovery through a centralized security layer for multiple consuming applications. It enforces data privacy policies and thus reduces risk to data privacy.
- Sustained funding – contributes to early big data project successes by reducing risk, and consequently accelerates funding for enterprise-wide adoption.
Access the following link to learn how one customer leveraged data virtualization to achieve a single, consistent view of the data across disparate systems to empower business users and drive big data adoption
- In and out of Blockchain - February 12, 2018
- A Virtual Sandbox for Data Scientists - September 11, 2017
- Growing Demand for Metadata-driven Integration Solutions – The Biggest Change in the 2017 Magic Quadrant for Data Integration Tools - August 24, 2017