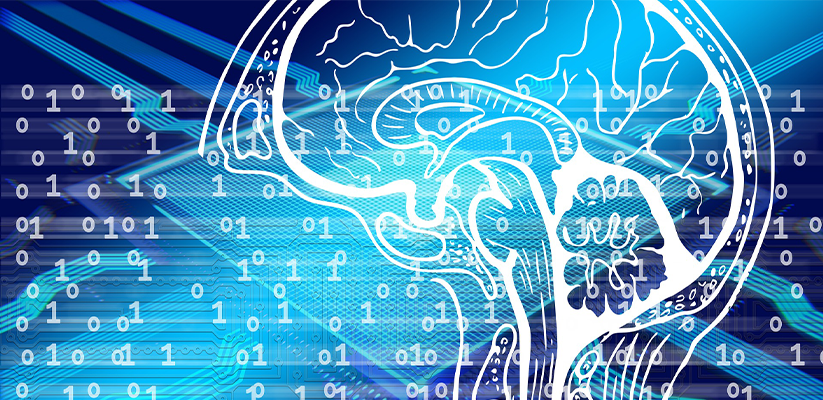
Having been privileged to witness the evolution of the data science and artificial intelligence (AI) scene in the Middle East for the past 10 years and having spoken at one of the first big data events in Dubai back in 2013, it is clear to me that there are considerable opportunities for AI in this vibrant region. Recently, I got the chance to present on the top 10 AI challenges of companies in the Gulf Cooperation Council (GCC) region, at Virtual Executive Boardroom: Key Insights on Becoming a Data-Driven Enterprise, which took place at DigiConnect (UAE) and was delivered to top C-level executives and senior data managers from the most relevant companies in the GCC region.
In this post, I will not get into each of these ten challenges. However, I will focus on a common issue that came up as a top priority for them in a quick live poll during the session: The issue of trusting decisions made by AI.
Trust Is at the Heart of the Deep Neural Network
Interpreting deep learning networks takes place in a tough playground, so making AI interpretable serves one specific goal, and that is to trust the decisions made by AI models. Is there no task as difficult as explaining a deep neural network? Any AI strategy is a cultural transformation project and not a technology project or a so-called digital transformation project. We evolved to predict the trustworthiness of other humans, and we learned to quickly decide to trust another person based on simple surface cues, or flag him or her as “deceptive.” This is why neuroscience research shows that we are less able to predict the trustworthiness of avatars or robots. As the clinical psychologist Doris Brothers states: “Trust rarely occupies the foreground of conscious awareness. We are no more likely to ask ourselves how trusting we are at any given moment than to inquire if gravity is still keeping the planets in orbit.” For this reason, we will increasingly question the trustworthiness of AI decisions as they percolate within companies.
How Can AI Leaders in the Middle East and Africa (MEA) Build a Culture of Trust?
An important trait for an AI leader is being an open business coach who intuitively understands the numbers. The role of such leaders is to use AI insights to foster a social learning and team sport culture. Coding is one the critical skills of data scientists, but not necessarily of the leader, as long as they can inspire curiosity in their teams.
Indeed, trust tremendously affects the delegation of work or decisions to robots. Trust in AI cannot become established if we apply traditional rules of the workforce regarding chain-of-command and information control. This is what Julius Caesar communicated to his army when he told his soldiers that he won’t march while ignoring their decisions. Caesar simply reminded them of who they were and trusted them to make the right decisions.
Here is another recent example from the Liverpool Football Club: Members of the data science department out there explains how Liverpool coach Jurgen Klopp is open to them and intuitively understands the numbers. He clearly mentions that they are the reason he got recruited at Liverpool. On the other hand, one of the players says: “But the manager doesn’t hit us with statistics and analytics. He just tells us what to do.” While many observers would have stated that Klopp failed on several occasions at Dortmund Club, AI showed different insights to the management. So for businesses, trusting that AI is the reason behind their success, and bestowing the same trust to their employees would enable true, explainable decisions to flow between AIs and humans across all value chains.
Explainable AI Systems
Here are 6 key attributes for an explainable AI:
- The Ability to Revisit AI Decisions: Introducing flexibility. HBR Research said that “Once we’ve made a decision to trust, we tend not to revisit it. That’s dangerous.”
- An Alert System: Alerting stakeholders of the AI model whenever it gives an output that falls beyond its domain of applicability.
- An Ambiguity Measure: Identifying the difference between an output of a decision and the explanation itself.
- A Similarity Measure: Measuring some kind of mutual information between the decision output and its explanation.
- A Cultural Measure (Delivered by a Champion or Leader): Analyzing the effects of the decision on a company’s culture and conveying the AI decision across value chains.
- An Incentive Measure: Increasing the benefits of a successful trust interaction of both AI and human and decreasing the cost of one that is unsuccessful.
An AI System Built on a Culture of Trust
In addition to understanding how their culture perceives trust, AI Leaders in the MEA region should also be able to foresee AI trust as something to be earned through consistent interactions and preventive measures like alert systems, which are more effective than the incentive measure in the MEA context. This is just one of the key pillars for a successful AI strategy.
My final message to MEA’s AI leaders or any AI expert pursuing a leadership role in their organization: You are the leaders of tomorrow; driving the company’s cultural evolution is more important than letting technology drive the evolution; if technology drives, your data/AI strategy will risk social resistance, and this is a risk that no leader should be willing to take in these uncertain and disruptive times.
- If Trust is the Main Ingredient of Leadership, Is Trust the Main Ingredient of Successful AI? - November 18, 2020
- The Main Pillars of Future Polymer Factories, the Next Future Material for AI - November 15, 2019
- From the Bronze Age to Today’s AI-based Circular Economy, and How Data Virtualization and Federated Learning Make It Possible - August 13, 2019