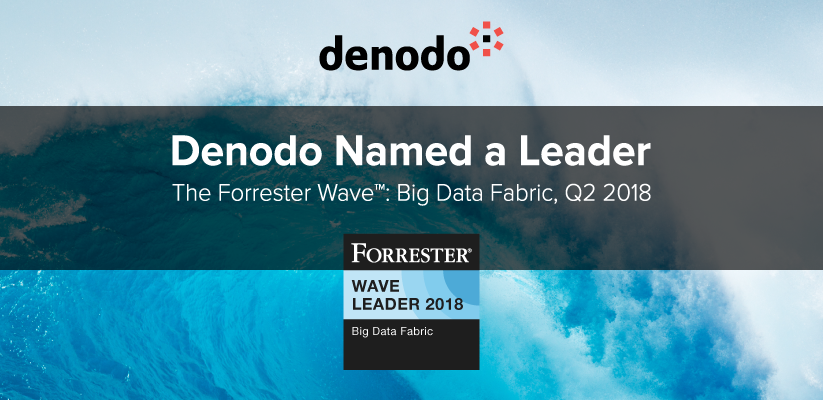
The recently published The Forrester Wave™: Big Data Fabric, Q2 2018 highlights the need for an integrated infrastructure – the ‘fabric’ – to support your big data. In the headlong rush to create data lakes and other big data architectures, organizations need to think about how they will manage their big data repositories and provide quick and easy access to their users. Without this, the repositories risk becoming ‘big data silos’ which further complicates the information landscape instead of simplifying it.
This is where the big data fabric comes into play. Forrester defines a big data fabric as a “platform [that] accelerates insights by automating ingestion, curation, discovery, preparation, and integration from data silos.” Obviously, you need to think about how you will fill your big data repository, but more importantly you need to think about how your users will find and access this data to gain those business insights.
Forrester have also created a conceptual architecture for the big data fabric below, sourced from their 2016 Big Data Fabric report by analyst Noel Yuhanna.
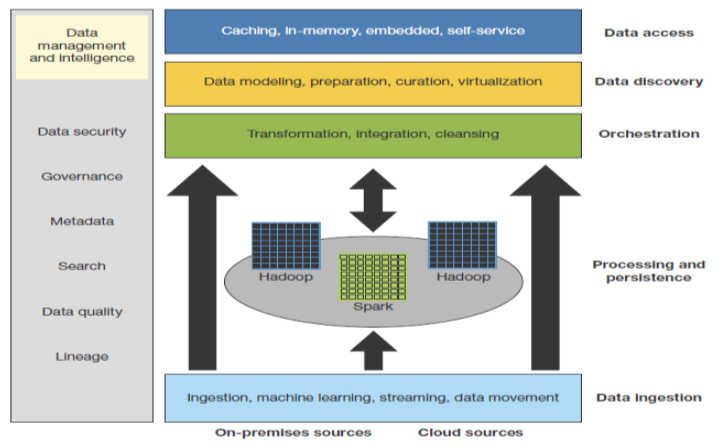
Figure 1: The Big Data Fabric Architecture Comprises of Six Layers
In this conceptual architecture, there is layered functionality i.e.
- A data ingestion layer to load data into the big data repositories (e.g. sqoop, flume, nifi, etc.);
- An ‘orchestration’ layer to integrate and transform the data into something meaningful and consumable by the users;
- A data discovery layer so that the users can discover what data is available for them to use;
- A data access layer so that the users can get the data that they need to obtain business insights;
- Finally, a data management and intelligence layer that is interwoven throughout the other layers to provide capabilities such as: security, metadata management, governance, and so on.
A key thing to note in Forrester’s big data fabric architecture is that the ‘processing and persistence’ layer has multiple parts. It is not a single repository or data lake; it is made up of multiple data stores and processing engines, each one ‘fit for purpose’ for a specific data set or computational workload. This is also something that I have noticed from discussions with a variety of organizations. Companies are not just building a single, consolidated big data repository (aka a ‘data lake’), instead they are building many of them for different purposes, in different divisions or business units and in different geographies, etc. Most organizations will have a number of big data repositories and this makes the big data fabric infrastructure even more important.
Now let’s compare this to Denodo’s data virtualization reference architecture:

Fig 2. Denodo Reference Architecture
You can see that the architectures are very similar. For example, in the Denodo reference architecture there is:
- A data ingestion layer (‘Data Collection’ on the reference architecture);
- A processing and persistence layer (‘Data Storage and Analysis’), however, this is more than just Hadoop-based, this layer also includes more traditional data storage and processing, such as a data warehouse;
- A data virtualization layer, which includes data discovery through the Data Catalog, data integration and transformation (with advanced performance optimizations for big data scenarios), data access as ‘data services’, and finally, data security, governance, lineage, etc.
Given the strong correlation between the capabilities required for Forrester’s big data fabric and those provided by a ‘best-in-class’ data virtualization platform, it’s no wonder that the Denodo Platform was ranked as a Leader in the Forrester Wave for Big Data Fabric. As the report notes, “Denodo’s key strength lies in its unified data fabric platform that integrates all of the key data management components needed to support real-time and dynamic use cases, such as real-time analytics, fraud detection, portfolio management, healthcare analytics, and IoT analytics.”
Companies such as Logitech, Autodesk, and Asurion are all using the Denodo Platform as a big data fabric, giving them the supporting infrastructure that allows their data analysts and business users to quickly and easily find and use the data that they are storing in their big data repositories.
- The Energy Utilities Series: Challenge 3 – Digitalization (Post 4 of 6) - December 1, 2023
- How Denodo Tackled its own Data Challenges with a Data Marketplace - August 31, 2023
- The Energy Utilities Series: Challenge 2 – Electrification (Post 3 of 6) - July 12, 2023