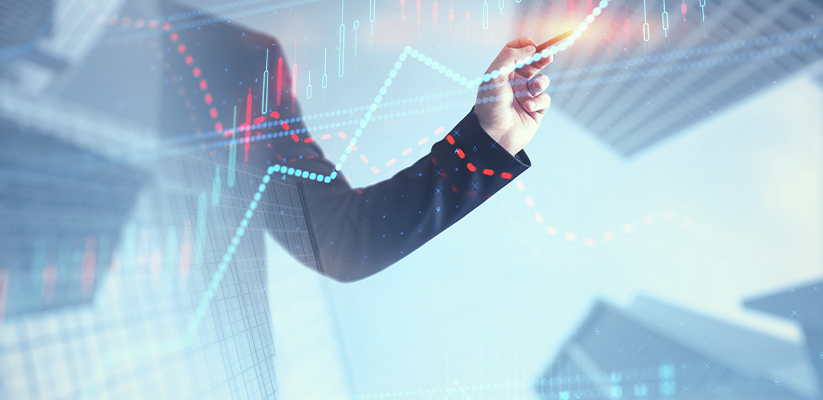
The data landscape has evolved and become more complex as organizations recognize the need to leverage data and analytics. Generative artificial intelligence has further put pressure on organizations to manage this complexity. At TDWI, we see companies collecting traditional structured data as well as unstructured data (such as text and images) to support more advanced analytics. Unpublished TDWI research in 2024 about data and analytics shows these diverse data types growing. Additionally, survey respondents say their top data management priority is to support sophisticated analytics, including predictive analytics, AI, machine learning, natural language processing (NLP), and other advanced analytics techniques. This ranks higher than moving to the cloud, deploying a data catalog, or strengthening security and privacy.
Supporting AI requires new algorithms, new platforms, and new skills and roles, together with support for different personas. Organizations should be aware of several trends, both to help manage the current complexity of the data environment and to prepare for what is coming next.
Trend #1: Generative AI Makes Organizations Realize Their Data Foundations Need Work
Generative AI refers to artificial intelligence systems designed to create new outputs, such as images, music, text, and other forms of media, based on the input data they are trained on. This corpus is often the internet, especially in the case of large language models (LLMs), a popular kind of generative AI (think ChatGPT). In the same unpublished research, TDWI found that although less than 10% of respondents have generative AI systems in production, the majority of organizations are exploring or experimenting with the technology. Popular use cases include chatbots and employee onboarding systems. Some organizations are even looking to front-end their data stores with generative AI for analytics.
As organizations move forward with their generative AI strategies, they often want to use their own company data. Many do not want to use public AI models as they don’t want those models to train on their private data, so they use private foundation models and try to build their own applications. However, problems often arise. In some cases, organizations realize that their data foundation is not well suited to AI. Data may be in silos, which makes it difficult to build an enriched data set for AI or to build AI applications that utilize data in context using techniques such as retrieval augmented generation (RAG). Their data may not be of high quality. TDWI routinely finds that only about half of those same survey respondents are satisfied with their data quality. With generative AI also comes the need to make sure all data types, both structured and unstructured, are governed. That governance foundation needs to be implemented as part of the data management journey.
Trend #2: Organizations Are Moving Towards Unified Platforms for Advanced Analytics
Even before the rise of generative AI, TDWI found that organizations wanted to unify their data to support their advanced analytics initiatives. AI-driven analytics often require an enriched data set that might consist of diverse data types—for example, text from call center notes in combination with traditional data from ERP or CRM systems, to determine if a customer is likely to churn. Organizations like the idea of data unification to capture and leverage emerging data types, achieve faster time-to-insight, make more diverse data available for analytics, and improve platform performance and scalability.
Unified platforms are becoming quite popular, and approaches to unification include both logical and physical architectures.
Logical data architecture enables organizations to view their data as if it is a single data source, even though it might be in multiple physical silos. A data fabric is one approach to a logical architecture. The data fabric maps and connects relevant application data stores using metadata to describe data assets and their relationships. Popular approaches include semantic layers and virtualization layers.
Physical data architectures involve the physical implementation of data management on various platforms. Some organizations want to centralize all their data on one platform to unify it. For instance, data lakehouses are becoming popular. They combine a data lake and a data warehouse to provide warehouse data structures and data management functions on low-cost platforms such as cloud object stores. These new platforms have blurred the distinction between the traditional data warehouses and data lakes.
At TDWI, we find that no one approach is currently winning out over the others. It may be that because it is almost impossible to centralize all data, the data fabric approach will be utilized by more organizations in the future as they need to continue to unify their diverse data.
Trend #3: Data Products Are Becoming More Common
Both anecdotally and in our surveys, we see more organizations embracing data products—assets derived from data. These data products run the gamut from simple cleansed data sets to industry-specific scores on certain topics (e.g., risk) to machine-learning model templates.
If data is a product, it must be trusted and of high quality or no one will buy it. In addition, someone must own the product and be responsible for it, typically a person on the business side of the house. TDWI has recognized the emergence of data product managers who own these data products. In a 2023 TDWI Best Practices Report survey, nearly 40% of respondents have designated specific individuals, roles, or functional groups with responsibility for productizing and/or monetizing the data and data-derived assets they own. Another 30% are planning to implement this role.
These data products are important because they represent a new way to think about and deliver governed data to multiple user groups. They can also help provide order to some of the complexity of the data management environment, especially if there is a way to offer these products to more users, such as through an internal data marketplace.
Trend #4: AI Governance and Responsible AI Can No Longer Be Ignored
Data governance always ranks at the top of the list of data management priorities in TDWI surveys, but that isn’t the case for AI governance. AI governance includes the strategies, processes, and tools to ensure that the outputs of AI are trustworthy, accessible only to authorized individuals, and in full compliance with relevant regulations. Responsible AI calls for a proactive approach to identifying and mitigating business, legal, and ethical risks to create trust and deliver tangible business value. It includes safety, fairness, explainability, sustainability, reliability, and privacy.
Governance of AI assets includes the ability to document, version, and monitor AI models in production to ensure they don’t become stale. It will also be important to ensure that the output of these models is explainable and that the models don’t hallucinate (a term that applies to generative AI models that produce incorrect output). This is a new area but one that is going to be critically important for organizations.
What Trends Make Sense for Your Organization?
Does your organization need generative AI, and can it build the necessary data foundation to support it? Although generative AI is top-of-mind for many enterprises, that doesn’t mean you necessarily need it or need it right now. It will be important for your organization to first determine if there is a business problem you need to solve that requires generative AI. At TDWI, respondents to our surveys are giving generative AI a higher priority than machine learning for 2024. This is interesting, given that many organizations have success with machine learning applications such as predicting churn, identifying fraud, and improving their understanding of customer behavior. Do not jump on the generative AI bandwagon because you think everyone else is using it. Yes, there is value to be had from generative AI, but it requires that the right infrastructure, funding, skills, culture, and governance are in place.
If you decide to move forward with a generative AI application, your organization will need to determine what data is needed for the application, locate the data sources, and decide whether they are easily usable. It can make sense to utilize a phased approach, through which you might first build a chatbot (if that is a business need) that utilizes a single data source (such as product information), and then expand it to include customer data. It will be important to consider the architecture and build governance into the process.