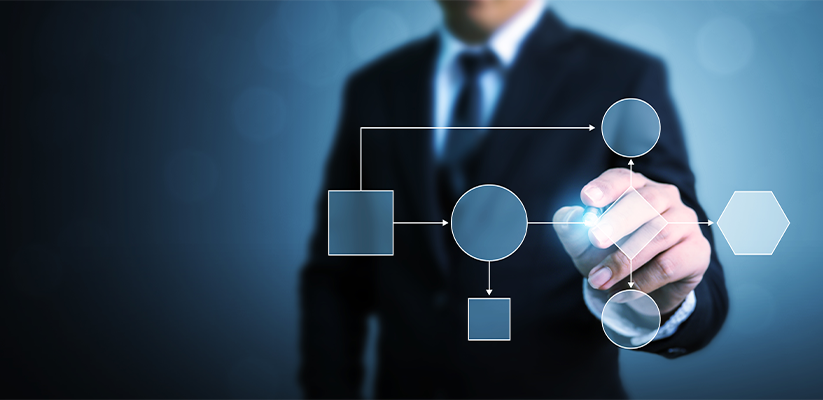
In 2024, generative AI (GenAI) has entered virtually every sphere of technology. However, companies are still struggling to manage data effectively, to implement GenAI applications that deliver proven business value. Gartner predicts that by the end of this year, 30% of all GenAI projects will not make it past the proof-of-concept (POC) phase, due to “poor data quality, inadequate risk controls, escalating costs, or unclear business value.”
This is hard to imagine, given the availability of modern data platforms like cloud data warehouses and data lakehouses. Developers are feeling pressure from the board of directors, the executive suite, and line-of-business managers to leverage GenAI to innovate and automate. Why are organizations still struggling with data quality, risk management, and cost management?
The Need for “AI-Ready” Data
The challenge is that, in order to experiment with a GenAI project, different business teams will pull data from wherever they can, often replicating it. This creates a data sprawl that can quickly get out of hand. Organizations need to know that the data they provide to large language models (LLMs) should be authoritative, accurate, and trusted. And if data is being replicated independently, there can be no data governance, and the data estate becomes the Wild West. AI developers and others are spending too much time finding and evaluating the quality of data, and not enough time using the available AI tools to generate business value. Organizations need a way to confidently supply their LLMs with AI-ready data, and they need to shorten the time to that data.
This is where logical data management comes in.
The Logical Approach to Managing Data
The logical approach is both simple and powerful: It enables fast access to data, without having to first replicate data. Organizations can implement a logical data management layer above their various data sources, including cloud data warehouses and data lakehouses, unifying the data as if it were within a common repository. Anyone from across the organization can leverage the same data for myriad different projects, formatted in different ways to support different needs.
A common data-access layer creates the foundation for seamless data governance and security, as well as the creation of a data fabric providing AI applications with trusted, AI-ready data from across the organization. It also enables financial operations (FinOps) capabilities, with which to easily manage costs across complex data landscapes.
Logical Data Management, a New Book from O’Reilly
O’Reilly is coming out with a new book, entitled Logical Data Management: An Essential Strategy for Transforming Your Business, which will answer all of your questions about what logical data management is and how it works. It will also cover how logical data management enables a wide range of use cases, from true data self-service, to advanced personalization, to “built-in” governance, risk, and compliance support.
Due to be published in August, a free, early-release preview is available now, which contains:
- The Preface, explaining how data management has challenges that pave the way for a logical data management approach
- Chapter 1, covering some of the key challenges with data warehouses, data lakes, and data lakehouses
- Chapter 2, defining logical data management through its basic architecture, introducing its benefits, and describing how it overcomes the challenges of existing data management technologies
A Complementary Preview
Download this free, early-release preview to learn how logical data management can greatly extend an existing data infrastructure. This is the necessary first step in gaining new levels of agility and the ability to innovate.
- O’Reilly Releases First Chapters of a New Book about Logical Data Management - January 21, 2025
- Active Data Architecture: The Need of the Hour - October 3, 2024
- Denodo Recognized as a Leader in Enterprise Data Fabric Evaluation by Independent Analyst Firm - February 29, 2024