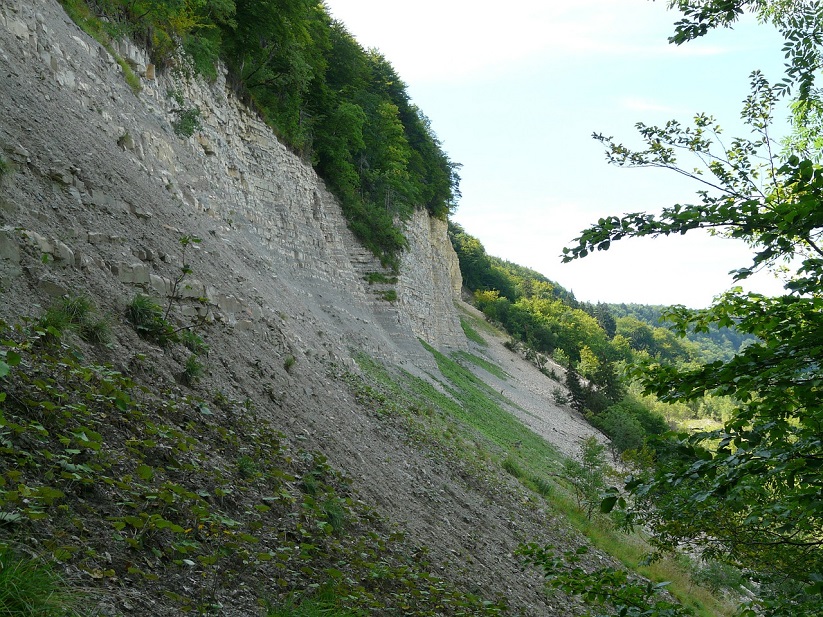
Landslides have caused more than 11,500 fatalities in 70 countries between 2007-2010. Over 1000 people were victims of a landslide that hit Sierra Leone in August 2017. The situation is getting worse as the volume and intensity of rainfall in West Africa is increasing. In April, Colombia’s landslide left at least 254 dead and hundreds missing.
Landslides are challenging across various levels, for example: social, economic, infrastructural, and environmental. They are triggered by a sudden and intense rainfall, anthropogenic activities, and hydrogeological factors. Although several efforts have been made in recent years to monitor and predict landslides, it is still a challenge to overcome.
Learning to read the signs
Currently real-time slope monitoring tools that utilize GPS and satellite data are being used to detect future landslides. These monitoring tools function with point-based sensors which are monitored from fixed positions; unfortunately this means that they are easily damaged, and need to be checked regularly. Furthermore these tools are not predictive and cannot provide an early-warning solution.
Predicting a landslide is an intense and immensely difficult operation because the signs are often too difficult to read and they occur without prior warning. This does not mean that the signs are not there however, it just means that haven’t learnt the best way to read them yet. And this is where the data plays a crucial role.
Predicting a landslide is a big data challenge
In order to predict and monitor landslides in real-time, several data sources are used including:
- Surface susceptibility
- Topography
- Soil type and vegetation
- Rainfall data
- Satellite data
- GPS data
- Magnetoresistive and optical fibre sensors
These data sources are located both within underground and overground sensors which affects the speed of data transfer latency, being much faster than overground sensors, making it more difficult in making decisions quickly.
The sheer amount of data coming from these diverse data sources are not only difficult to integrate, they are difficult to interpret due to differing aggregation patterns.
NASA taking a giant leap in their use of data
NASA has been working on the issue of data collection. One of their initiatives include the Global Landslide Catalog (GLC). GLC identifies rainfall-triggered landslide events around the world, regardless of size, impact or location. The GLC monitors the media, disaster databases, scientific reports, or other sources and provides it in its open data portal.
Another initiative by NASA is the GPM (Global Precipitation Measurement Mission) which uses satellites to quantify when, where, and how much it rains or snows around the world and also provides quantitative estimates of microphysical properties of precipitation particles.
Data virtualization: A technology to mashup data sources in real-time
Integrating these myriads of data sources is a key challenge to build a system that can predict landslides in real-time. Data virtualization is an agile data integration method that simplifies information access. Instead of using traditional data integration approaches such as data consolidation via data warehouses and ETL, or data replication via ESBs and FTP, data virtualization queries data from diverse sources on demand without requiring extra copies. Thus, you can run fast dynamic querying across your data from one source. In this case, we can solve the issue of data latency when processing sensor data in real-time since there is no data expiry issue.
Structured data like spatial and temporal data, NASA rainfall GPM data, and satellite can be mashed-up with other sources like semi-structured optical fibre sensors(the latest technology used for landslides). The latter act as a distributed nerve system and have the ability to detect a change of one centimetre over a distance of a kilometre. It can also measure and track early pre-failure soil movements.
Data virtualization could also integrate these sources with unstructured data coming from phone, radio, social media, email and text messages. With that vital information, authorities can act accordingly by dispatching ambulances or sending out an alert on Twitter about blocked streets and alternative routes, for example. For this command center, authorities also build landslide hazard GIS maps for either evacuation zones or to train historical landslide data using SVM machine learning algorithms to recognize areas of potential hazards.
In order to predict a landslide, you need to be able to read the signs. Without data, landslides might appear to the untrained eye as random occurrences with no prior warning. However, we have moved beyond this train of thought, as proved by the advances in data usage by the likes of NASA, and it’s now a case of finding the best solution to be able to read and comprehend landslide data and thus, act accordingly. Data virtualization, in this instance, acts as a means by which to decipher such data.
- If Trust is the Main Ingredient of Leadership, Is Trust the Main Ingredient of Successful AI? - November 18, 2020
- The Main Pillars of Future Polymer Factories, the Next Future Material for AI - November 15, 2019
- From the Bronze Age to Today’s AI-based Circular Economy, and How Data Virtualization and Federated Learning Make It Possible - August 13, 2019