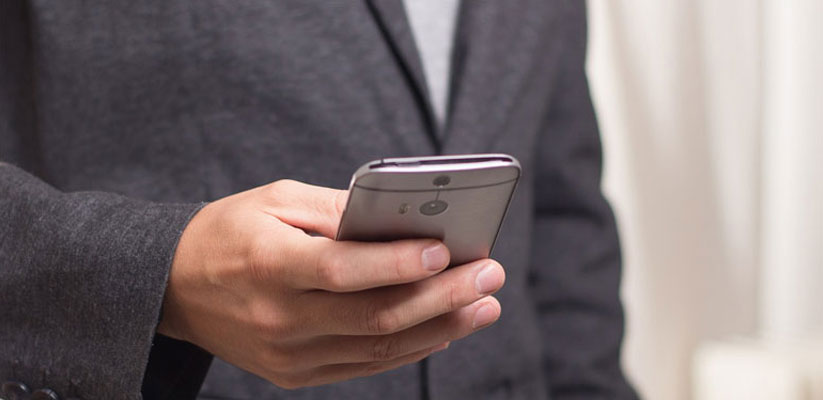
I recently attended Business Technology Forum by Forrester Research in Florida. As the event title indicates business and technology cross-over more than ever, just as software-as-a-service (SaaS) applications have done for operations, and now self-service business intelligence (Self-Service BI) and data exploration have taken off.
Gartner’s IT Glossary describes self-service business intelligence as end users designing and deploying their own reports and analyses within an approved and supported architecture and tools portfolio. The latter part of that statement includes provisioning of agile access to data. This is the role that IT plays in supporting self-service BI users who now have the freedom to design and build their own reports and analysis, while freeing IT to do more important things, thus benefiting both.
While provisioning of data for Self-Service BI is important, simply enabling self-service access to sources data systems can be problematic for many reasons. Firstly, security and monitoring have to be implemented in the data sources and cannot be flexibly managed. Secondly the performance and impact on source systems can be unpredictable. Thirdly, different users can interpret metadata and apply different data transformation rules yielding inconsistent interpretations of the data. Fourth, not all data sources can be provisioned directly to end users who may not know how to manipulate mainframe data or unstructured data. Finally, many self-service BI tools copy the data into their own memory or data stores before analysis which creates redundancy and higher costs in data storage and governance. These are not just challenges that may inhibit Self-Service BI, but also create data security and governance nightmares for IT.
Claudia Imhoff and Colin White point out in their TDWI article on Self-Service BI that IT’s role is to provision easy data access and this is best done through business-oriented, canonical data services. This is where Data Virtualization (DV) fits in and adds value to both the IT and business by creating a semantic layer that can address all of the above problems and provide additional leverage. DV can create abstraction layer that exposes heterogeneous data sources in normalized form such as a relational database table or XML or RESTful service. It also enables new paradigms of interaction with the data such as search and browse in addition to traditional query languages. Virtual integration can actually improve performance while minimizing replication … this is done through hybrid federation, that mixes advanced query optimization, caching and selective batch. Some BI tools will have a semantic layer of their own but there are at least two major advantages to having an independent data virtualization layer –one is that it is accessible to multiple BI tools and other applications and second is that DV tools offer much more sophisticated performance, security, data services publishing and governance features.
In summary, business users have good reason to want to explore data, build analytical sand-boxes, conduct their own iterative what-if analytics, and even share BI reports with their peers. Management and IT should encourage such Self-Service BI by enabling easy access to data, securely delivered through a data virtualization layer.
- Data Governance in a Data Mesh or Data Fabric Architecture - December 21, 2023
- Moving to the Cloud, or a Hybrid-Cloud Scenario: How can the Denodo Platform Help? - November 23, 2023
- Logical Data Management and Data Mesh - July 20, 2023
Suresh, we spoke today…
on this Self service BI vs DV…
in a batch mode, self service BIs can scan TBs of data… but with DV, with out data replication, and querying over network, what is the typical data size that denodo can scan & report , say in 5 -10 secs time?.