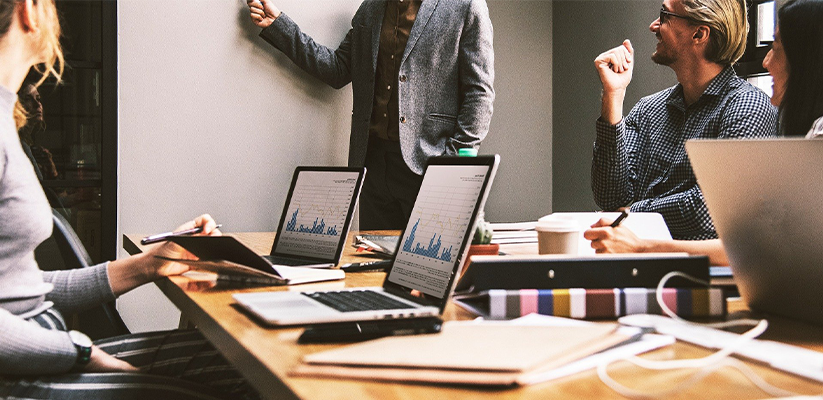
Learn in 12 minutes:
- What makes a strong use case for data virtualisation
- How to come up with a solid Proof of Concept
- How to prepare your organisation for data virtualisation
You’ve already read all about data virtualisation and you’ve decided: this is the right choice for your company. But how do you choose the right data virtualisation platform? And what do you need to bear in mind to make data virtualisation a success? This isn’t just about technology, but your business objectives too, and the way your organisation works. In this blog article, we’ll take you through it step by step.
Data virtualisation: what’s it about?
Let’s first take a look at why data virtualisation is so attractive. Because you’re placing a virtual layer over your data architecture, you can integrate your data from all your (source) systems and databases quickly and flexibly. You don’t have to copy or migrate any data, and you don’t need to worry about different information definitions either. Instead, you can rely on a single version of the truth. And while you are keeping your data under control efficiently via a single platform, users everywhere in your organisation will have easy access to the data they need to meet their specific information requirement via virtual tables – in a way that works best for them. This benefits the productivity of both IT and the business.
Securing data access is also controlled centrally with data virtualisation, and you always know where data is coming from. This transparency also helps your organisation meet the requirements for internal and external audits and regulations.
Use cases for data virtualisation
With the advantages we just mentioned, data virtualisation helps you tackle challenges in various situations. We’ll give you a few examples of strong use cases in a range of fields:
- Migration
Consider a use case like system migration: switching from a classical CRM system to a cloud solution. Or a phased transfer of legacy systems to the cloud. With data virtualisation, you can do this without operational downtime or stopping reporting. - Agile BI
With data virtualisation, you can use your data for governed (regulated) and self-service BI, as well as for data science and API or system links. Moreover, it’s ideal for ‘agile’ BI, whereby you’re producing dashboards and reports in very short cycles of tests, pilots and production. Would you like to connect SaaS cloud applications such as Salesforce or Google Analytics to your existing BI stream as new sources? You can! With data virtualisation, you’re combining all your data, even in a hybrid landscape. And you don’t have to worry about security either – after all, that’s controlled centrally. - Accessing (real-time) data
Is a source system not performing well in (near) real-time accessibility of large quantities of data and are your SLA agreements under pressure? With data virtualisation, you can ‘offload’ historical data to a different source and combine it with real-time data from the source system. Through querying systems more intelligently or caching optimisations, you can avoid overloading your source systems too. Even near real-time analytics on big data are possible, without first copying all manner of data with ETL processes. And you can combine an old data warehouse with a new data source into a virtual data mart without problems.
Developing use cases into a Proof of Concept
Purchasing and implementing a data virtualisation platform is no small job. It’s a major investment and a decision that reaches the core of your data architecture. That involves some radical changes. As always, a Proof of Concept will help you make the best choice of solution. Thorough preparation is crucial to that. First off, you need to think carefully about the use cases you want to develop in your Proof of Concept. What are the possibilities for bringing about improvements with data virtualisation? The next step is describing the use cases properly. Which processes (or parts of processes) within the organisation are involved? Where do they start and end? And how do they deliver value for the organisation?
Mapping out expectations and requirements
After describing the use cases, the next job is to make an inventory of your stakeholders’ expectations and requirements. These are all the parties involved in the decision-making process who will shortly be presented with the chosen data virtualisation platform. Be sure to include the business and IT, but don’t forget to map out the needs and wishes from architecture, governance or e.g. purchasing too. You can make this inventory by means of interviews or workshops, depending on the possibilities. Once you’ve put together all the expectations and requirements, you can translate those into ‘Proof Points’. These are the aspects and properties that you want to demonstrate in your Proof of Concept. So, these are the Proof Points by which you’ll evaluate the data virtualisation solution.
Linking Proof Points to use cases
Don’t limit yourself when nominating Proof Points. It’s better to have too many or even conflicting Proof Points at first than to overlook aspects. All of that will be taken care of later on when classifying the points. That’s when you can cross off any duplicate Proof Points, as well as those that conflict with each other or with your data architecture, from your list. You’ll also evaluate the various aspects or properties for their value – some will be more important than others. This will give you the Proof Points that you actually want to turn into reality in your Proof of Concept.
You link the Proof Points you retain to your inventory of use cases. Make sure you ‘cover’ all the points when substantiating your use cases, but minimise the number of use cases. The aim of your Proof of Concept is to demonstrate that the data virtualisation platform you want to choose is suitable. That means your focus needs to be on the Proof Points, not on the use cases themselves.
Points that apply for all use cases
Alongside the Proof Points for your specific use cases, you’ll also have to deal with matters that are important for any data virtualisation application, in any situation. Examples of these kinds of aspects are security and performance, which we’ve already talked about. But there are more general points that you should test the Proof of Concept for your data virtualisation platform against too. These include:
- Diversity of data sources
Your data virtualisation platform needs to be able to access all the source systems and technologies in which data are stored. - Diversity of data access
Support for all the APIs, languages and file formats that data users will query and through which you supply data to them is essential. - Data transformation
It needs to be possible to radically transform data from your data sources before sending it to your data users. Examples of transformations are filters, calculations, aggregations and integrations. - Data management
You’ll want to be able to manage and monitor the data virtualisation platform, along with all the defined specifications. This means you’ll need features like data lineage analyses, impact analyses, comprehensive and detailed logging and defining authorisation and authentication rules. - Ease of documentation and searching
Your data virtualisation platform needs to be able to work together with your existing data catalogue for documentation or to be able to serve as an integrated data catalogue for all defined objects. It’s important that you can add concepts to this catalogue and that all the documentation is searchable. This enables users to search for suitable data for their reports themselves. - Database server independence
It needs to be possible to work with a range of databases, on-premise and in the cloud, such as Microsoft SQL Server, Oracle and PostgreSQL, as well as Snowflake and Azure DB. You don’t want to be limited as to what new databases you can introduce in the future either. - Query optimisation
Support for an advanced query optimiser ensures good performance. Ideally, you’ll opt for a cost-based query optimiser, which takes account of data properties when executing a query and supports these technologies for efficiently linking multiple sources. Transparency is another prerequisite, so a developer can see in detail how a query is being executed. - Caching
You need to be able to cache the query results built within your data virtualisation platform. This means that the software physically stores this data for reuse. This is sometimes necessary to make sure that the data sources aren’t under too much load and improve performance or to demonstrate a consistent data result over a certain period. - Query pushdown
In order to make optimum use of your database’s processing power, you’ll want queries received by the data virtualisation platform to be forwarded on to the underlying databases(s) as far as possible. This is called query pushdown. - Local support and local clients
It’s important that the data virtualisation platform you choose is supported in your country, by the supplier itself or by a consultancy organisation. Also look to see whether there are any other clients in your country using the solution, and whether the supplier considers this country to be a mature market. - Maturity
Investigate whether the data virtualisation platform has proven its worth for other clients. It needs to be robust and you need to be able to rely on it.
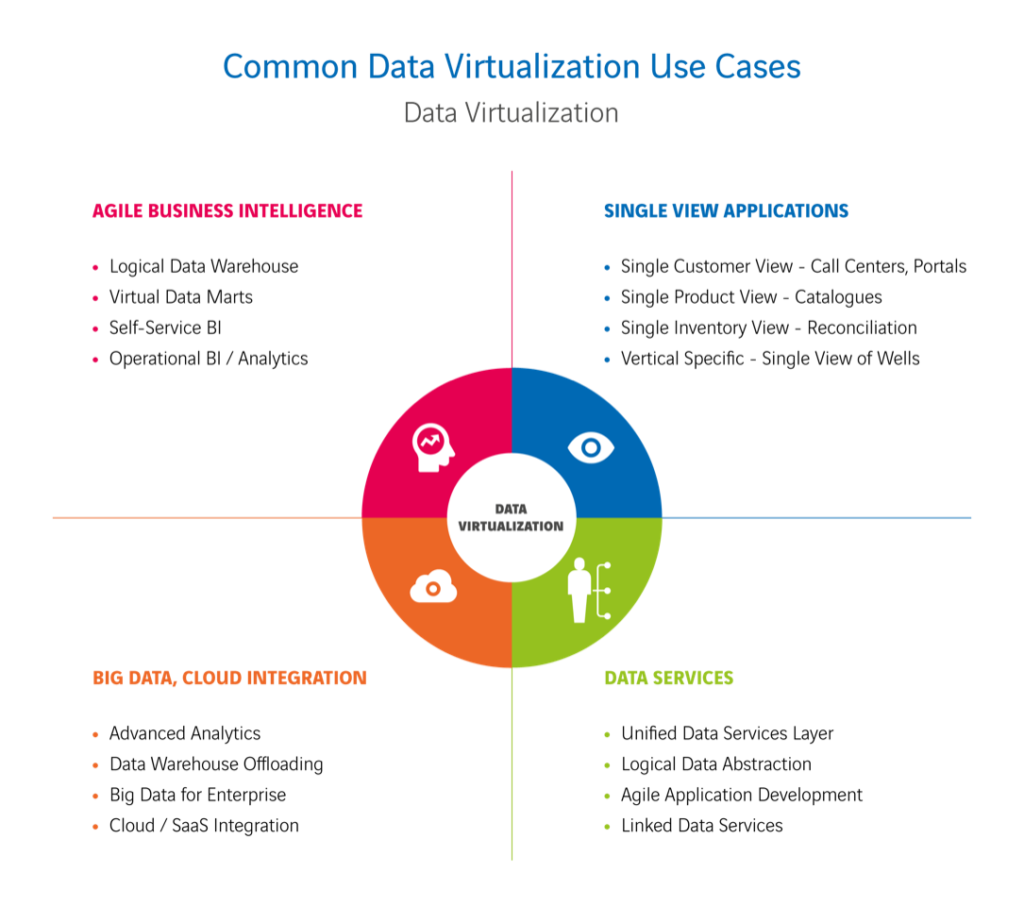
Different working method needed
Have you chosen the ideal data virtualisation platform based on your Proof of Concept? Then you might think you’re all done, and that the success of your data virtualisation plans is assured. But ultimately, that success will be determined by some very different matters as well. If you carry on doing things the way you used to, you’ll get just what you got before. In other words: the big question is whether introducing data virtualisation enables you to adapt your working method to it! We’ll lay out the most important points for consideration for you:
- Opt for agile development and deployment
Data virtualisation demands an agile working method, no complex OTAP procedures or waterfall methods. You’re playing the game of development and deployment in a cycle of Testing, Pilot, Production. A precondition for successfully ‘walking the agile talk’ is that you’ve got all the various activities under control that there’s a balance between them. Only that way will you really start developing more quickly and adopting new functionality. - Local direction and decentralised freedom
You won’t be used to it, but it really is what happens: with data virtualisation, your users can get to work on data themselves. Do marketing, data science or the actuaries at your company want to try something out, for example? Then they won’t have to go to the IT department to ask for a new data mart, but can instead experiment with data independently in virtual tables that they themselves compose. Even adding data from their own sources is possible there. You can give them this freedom with peace of mind, because with data virtualisation, your users aren’t changing your data. Furthermore, data and security governance are centrally controlled, and the origin of data is always fully transparent. That means you don’t need to worry about data integrity, incorrect interpretations or compliance. - Keep moving
The shift to the cloud, system migration, an outdated data architecture. There are continual changes, and you can’t prevent them. So, stop looking for that one product that solves everything – you’ll never win that race. With data virtualisation, you’re disconnecting your sources from your data usage. That allows you to migrate step by step and keep moving at a suitable pace, whereby you’re supporting your organisation instead of pushing technology. - Think in layered solutions
Don’t try to merge as many data transformations as possible in one go. It’s best to think in layered solutions with stacked virtual tables in order to maximise the possibilities for reuse and adaptation of data and data structures. For traditional ETL thinkers, that might seem like a performance nightmare, but you really don’t have to be afraid of it. In practice, your dataset won’t be going through all those stacked views – instead, those stacked views will be highly efficiently rewritten and simplified into queries to the sources.
The moral of this whole story? It’s important to choose the right data virtualisation platform with a decent Proof of Concept, but even more important is the realisation that you need to change as an organisation in order to benefit from the advantages of data virtualisation. Do you want to do that? And are you able to? You need to try that out first before you choose too.
How is your organisation making the best use of data virtualisation?
Jonathan Wisgerhof and Allard Polderdijk explain how to choose the right data virtualisation platform and make sure your organisation is ready for it!
How do you choose the right data virtualisation platform? And how do you prepare your organisation? Jonathan and Allard explain it all!