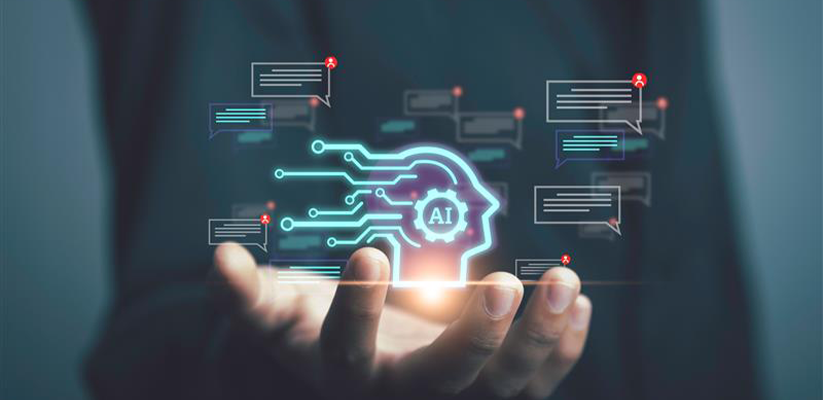
The rapid advancement of technology in recent years has dramatically transformed the landscape of enterprise operations. This transformation can be encapsulated in the evolution of the Agentic AI Model as defined by Carmen Di Nardo in “From deterministic robots to intelligent agents: how AI is improving process automation.” The Agentic AI Model has undergone four distinct phases: robotic process automation (RPA), cognitive automation, digital or intelligent assistants, and the current phase, agents, powered by generative AI (GenAI). Each phase represents a significant leap forward in automation, AI, and the use of data, enabling enterprises to function more efficiently and achieve accelerated outcomes.
Phase 1: Robotic Process Automation (RPA)
RPA involves the use of bots to automate repetitive, rule-based tasks that were traditionally performed by humans. These tasks often include data entry, transaction processing, and simple data manipulation.
Key Features:
- Automation of repetitive tasks
- Reduction of human error
- Cost savings and increased efficiency
Horizontal Use Cases:
- Finance: Automating reconciliations, processing invoices, and managing accounts payable/receivable
- Human Resources: Automating payroll processing, employee data management, and leave approvals
- IT Operations: Automating routine maintenance tasks, software updates, and system monitoring
Vertical Use Cases:
- Healthcare: Automating patient data entry, claims processing, and appointment scheduling
- Manufacturing: Automating inventory management, order processing, and supply chain logistics
- Retail: Automating order fulfillment, returns processing, and customer data management
Phase 2: Cognitive Automation
Cognitive automation marks a significant advancement in process automation by leveraging artificial intelligence and machine learning to enhance adaptability and decision-making. This approach goes beyond traditional rule-based automation by interpreting unstructured data such as emails, documents, and images, and continuously learning from historical and new data to adapt to feedback.
It is particularly effective in predictive tasks like demand forecasting, for which it analyzes past patterns, seasonality, promotions, and economic conditions, to guide business decisions on production planning, inventory management, and resource allocation. By continuously training with new data, these systems improve over time, providing more accurate and efficient forecasts and supporting more complex processes with a higher level of autonomy. This results in more informed business strategies and the ability to more flexibly adapt to market changes, ultimately enhancing the efficiency and accuracy of a variety of business operations.
Phase 3: Digital or Intelligent Assistants
This phase builds upon RPA and Cognitive Automation by integrating them with workflows and adding a layer of AI. These intelligent assistants can handle more complex tasks, make decisions based on predefined rules, and provide support in a variety of business processes.
Key Features:
- Integration of RPA with workflows
- Enhanced decision-making capabilities
- Improved process efficiency
Horizontal Use Cases:
- Customer Support: Intelligent chatbots providing 24/7 support and resolving common queries
- HR Processes: Automating employee onboarding processes, including document verification and induction scheduling
- IT Support: Automating routine IT tasks like password resets and system diagnostics
Vertical Use Cases:
- Healthcare: Virtual assistants for patient triage, appointment reminders, and follow-up communications
- Manufacturing: Intelligent assistants for production monitoring, quality control, and equipment maintenance
- Retail: Chatbots for customer service, personalized shopping experiences, and inventory management
Architecture:
In the example use case illustrated below, RPA, AI, and data work together to enhance the functionality and efficiency of a contact center. RPA automates repetitive tasks such as data entry and process execution, enabling the contact center to efficiently handle high volumes of routine inquiries. AI, particularly through Google’s Dialogflow and Contact Center AI, processes and understands user inputs, detects intent, and generates appropriate responses using natural language processing.
The AI components, including virtual agents and agent assist tools, leverage real-time data from knowledge bases and backend systems to provide accurate, relevant information. Data serves as the foundation for these operations, feeding the AI systems with the necessary context and information to perform tasks, generate insights, and support decision-making processes in real time, so that both automated and human agents can deliver high-quality service to users.
Phase 4: AI Agents Driven by GenAI
The current phase of the Agentic AI Model, driven by GenAI, marks a significant shift towards creating autonomous AI agents. These agents not only automate and streamline processes but also generate new content, ideas, and solutions while making independent decisions with minimal human oversight. By leveraging advanced automation, workflows, and low-code/no-code platforms, organizations can develop AI agents that drive accelerated outcomes across various enterprise functions, from call centers to marketing and HR. Their enhanced autonomy enables them to adapt to changing environments and provide intelligent support, revolutionizing operations and redefining human-machine collaboration.
Key Features:
- Advanced automation and AI integration
- GenAI for content and solution creation
- Low-code/no-code platforms for ease of deployment
- Enhanced efficiency and accelerated outcomes across enterprise functions
Horizontal Use Cases:
- Marketing Campaigns: AI agents creating personalized marketing content and strategies
- Call Centers: AI agents handling complex customer interactions, resolving issues, and providing insights to human agents
- HR and Recruitment: AI agents conducting initial interviews, assessing candidate suitability, and streamlining the hiring process
Vertical Use Cases:
- Healthcare: AI agents for personalized patient care plans, predictive diagnostics, and telemedicine consultations
- Manufacturing: AI-driven predictive maintenance, process optimization, and supply chain forecasting.
- Retail: AI agents for dynamic pricing strategies, personalized customer recommendations, and trend analysis.
Integration of Vertex AI and the Denodo Platform in Agentic AI
Data serves as the lifeblood of Agentic AI systems, playing a crucial role throughout their lifecycle and significantly impacting their success. These autonomous agents rely on vast amounts of high-quality, diverse data to perceive their environment, reason about complex situations, and make informed decisions. From the initial training phase to continuous learning and adaptation, data fuels the AI’s ability to understand context, recognize patterns, and generate insights.
In the life sciences industry, for example, domain-specific data is critical for effective functioning, whether for patient monitoring, drug discovery, or healthcare professional engagement. The quality, relevance, and comprehensiveness of the training data directly influence the AI’s performance and the trust placed in its outputs. Moreover, the integration of AI agents with enterprise systems enables them to tap into a wealth of data sources across organizations, breaking down silos and enabling smarter, data-driven decision-making. As Agentic AI systems continue to evolve, their ability to process, learn from, and act upon diverse datasets will be paramount in realizing their full potential and driving innovation across a variety of industries.
Data Ingestion and Preparation:
- The Role of Denodo: The Denodo Platform acts as a crucial data layer, aggregating data from multiple sources into a single, coherent dataset. This data is then pre-processed and transformed as needed, providing high data quality and consistency.
- Benefit: This unified data can then be fed into Vertex AI for model training and inference, so the AI models are trained on comprehensive, accurate datasets.
Model Training and Deployment:
- The Role of Vertex AI: Utilizing the aggregated data from the Denodo Platform, Vertex AI simplifies the model training process with its AutoML capabilities or custom training environments. Once trained, these models can be seamlessly deployed and scaled within the Vertex AI ecosystem.
- Benefit: This integration keeps models continuously updated with real-time data from the Denodo Platform, enhancing their accuracy and relevance over time.
Operationalization and Automation:
- The Combined Roles of the Denodo Platform and Vertex AI: After deployment, the Denodo Platform continues to provide real-time data integration, while Vertex AI manages the operational aspects of the AI models, including monitoring, updating, and scaling.
- Benefit: This continuous flow of data and model updates enables Agentic AI systems to operate autonomously, making informed decisions based on the latest information.
Phase 5: A Paradigm Shift in the Agentic AI Model
- Generative and Autonomous Creation: AI agents can independently generate new content, ideas, and solutions, moving beyond predefined rules.
- Integrated Automation and Self-Management: Combining GenAI with advanced automation enables seamless operations, with agents autonomously managing processes.
- Low-Code/No-Code Platforms: These platforms enable non-technical users to build AI solutions, while agents can self-configure for a variety of different applications.
- Enhanced Autonomous Decision Making: AI agents analyze data in real time, making informed decisions with minimal human intervention.
- Resource Augmentation and Self-Direction: They can autonomously handle complex tasks, freeing human employees to focus on higher-value activities.
- Adaptive Learning and Self-Improvement: Agents learn from experience to continuously enhance their performance without explicit reprogramming.
- Autonomous Problem-Solving: Advanced AI agents independently identify issues and implement solutions, showcasing robust problem-solving capabilities.
Architecture:
Conclusion
The evolution of the Agentic AI Model from RPA to Digital Assistants, and now to AI agents driven by GenAI, represents a remarkable journey of technological advancement. Each phase has brought about significant improvements in efficiency, decision-making, and the overall functioning of enterprises. As we continue to embrace and innovate with AI, the future holds even greater potential for transforming how businesses operate and achieve their goals.
This phase also enhances a customer’s journey towards enhancing the robustness and agility of their integrated AI and data management systems.
By leveraging the synergistic capabilities of Google’s Vertex AI and the Denodo Platform, we have laid a strong foundation for advanced, scalable, and intelligent operations.
This phase has not only demonstrated the seamless orchestration and grounding of AI agents but also underscored the critical role of unified data access in driving accurate and relevant AI outputs. Moving forward, the insights and efficiencies gained in this phase will propel us towards even greater innovation and operational excellence, so that our systems remain at the cutting edge of technological advancement and business value delivery.
By understanding and leveraging the capabilities of each phase, enterprises can better navigate the complex landscape of automation and AI, so they can remain competitive and forward-thinking in an ever-evolving digital world.
- The Evolution of the Agentic AI Model: From RPA to AI Agents - November 12, 2024
- Breaking Boundaries: Denodo and Snowflake Pave the Way for Advanced Enterprise AI - May 30, 2024
- Enterprise Data Unlocks Generative AI Potential with Vertex AI + Denodo - April 8, 2024
- The Evolution of the Agentic AI Model: From RPA to AI Agents - November 12, 2024