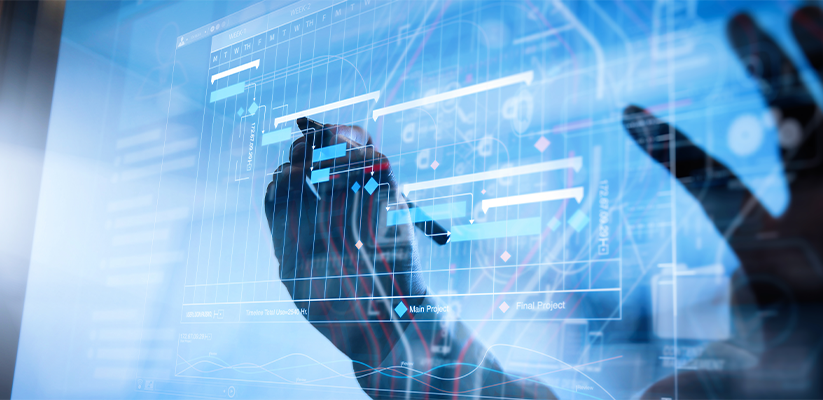
In today’s data-driven world, the power of comprehensive enterprise data solutions is undeniable. Even smaller data projects can help empower organizations to efficiently harness data for informed decision-making. In this blog post, to ensure that you can unlock the full potential of data, I’ll offer my top-seven best practices for implementing any enterprise data solution.
Data teams tend to focus on the technical best practices around the use of the tools and products selected for new data projects, but all too often overlook the larger business planning aspects of the project. This can leave even the most well-designed technical solution set up for failure, or even worse, apathetic disuse. Though nothing can replace the value of an experienced project manager’s involvement, these practices can help set up a data project for success.
- Consider the Interplay of Consumption and Discovery Mechanisms
The success of any data solution hinges on the synergy between data discovery and consumption.
To maximize efficiency:
- Determine the tools that data consumers will use to discover data. Isolate the distinct needs of different consuming users, such as business users versus mobile app developers.
- Streamline the transition from data discovery to utilization.
When these aspects are seamlessly interconnected, a data solution becomes more user-friendly and efficient.
- Define a Pilot and Communication Plan Early
Project rollout schedules are sometimes presumed when they should be far more critically prescribed.
Plan early for the success of the project by:
- Defining a manageable pilot to evaluate the solution, including contingencies to pivot timelines based on outcomes
- Ensuring agreement on communication timelines, content, and responsibilities
- Communicating the business value of the data projects early to the targeted consumers and involving them as appropriate in the design and pilot
- Maintaining recurring communications to increasingly larger groups of consumers, with progress updates and feedback mechanisms
- Document Clear Usage Definitions
Clarity is essential. Ensure that every data element has a documented usage definition, answering the questions like the following:
- Is the data appropriate for the decisions users need to make?
- Can users confidently – and legally – share this data?
These definitions not only enhance the quality of decisions but also mitigate the risk of data misuse.
- Implement Strong Governance
Robust governance is paramount for maintaining data integrity, accuracy, and security, whether the project is driven by business-user self-service models or tighter war-chest approaches.
Address the following key data governance questions:
- How can consuming users trust the data provided?
- How can data owners be assured that the data is being used appropriately?
- Which assets require a request-and-approval process?
It is essential to create a structured, secure data environment.
- Design for Bounded Domains
Organizing data assets according to specific domains creates a focal point that minimizes interdependencies. This is far more effective than organizing around such technical details as source-system requirements. For best results, consider processes, organizational structures, and data domains. This aligns data with how the business operates, which will ultimately streamline data access.
- Establish a Strong Monitoring Practice
Monitoring goes beyond measuring adoption and ROI.
Monitoring is also vital for:
- Auditing governance compliance
- Detecting issues early
- Assessing the need for deprecating or implementing new data assets
A robust monitoring practice keeps data systems aligned with organizational goals, enabling you to more accurately plan for future needs.
- Plan for Continual Improvement
To keep your data solution relevant and effective, plan for continual improvement by implementing a two-way communication channel enabling data consumers to report issues, ask questions, and request new assets. This type of feedback mechanism is vital for the ongoing evolution of any data solution.
Develop a strong testing practice that adapts to changing demands. Even well-designed assets cannot predict every usage pattern or change in data scope, so testing is essential to maintain not only data accuracy and security, but usability and relevance.
Move Forward with Confidence
Incorporating these seven best practices can help to ensure your data solution not only meets the current needs that drove its innovation, but also provides the underlying building blocks of a strong data practice that are so often overlooked. By implementing such practices at the outset of a project, your organization can be better equipped to adapt to an ever-evolving data landscape. Every data solution is a crucial investment in an organization’s future, making data-driven decisions more informed and accessible than ever before.
- Top 7 Business Best Practices for Data Projects - January 11, 2024
- Data Management Trends in 2023: My Top 5 Trends to Watch - January 25, 2023
- Data Mesh vs Data Fabric: Understanding the Key Differences (2023) - January 17, 2023