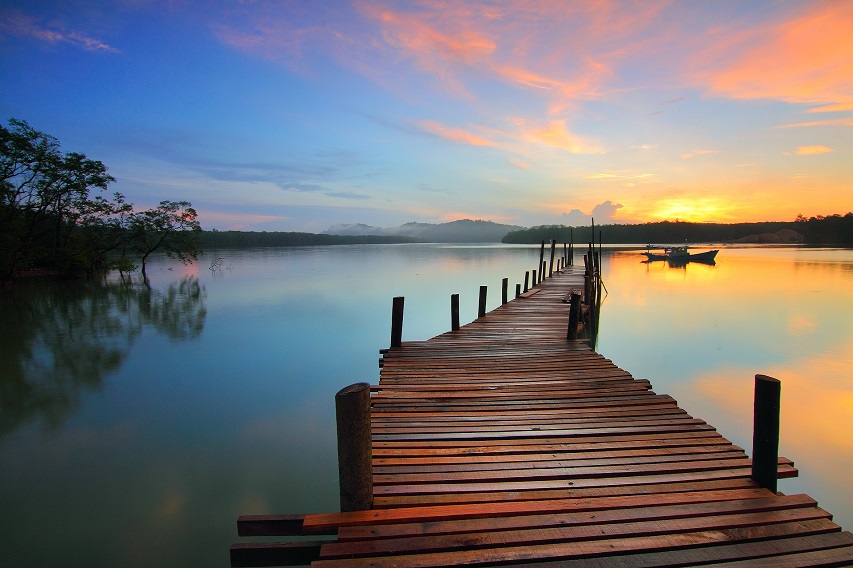
With data becoming the brain food to the intelligence of every organization, regardless of size or sector, it has become crucial to harness this data to achieve the best results, make the most informed decisions and improve productivity. However, with every action, reaction and interaction a fresh load of data
Managing the Avalanche
It becomes key to store and
The benefits of a data lake are threefold:
- They make data discovery easier
- They reduce the time spent by data scientists on selection and integration
- They provide massive computing power, allowing data to be efficiently transformed and combined to meet needs of any process that requires it.
A recent analyst report confirmed the success of the data lake discovering that those employing this architecture were outperforming their peers by 9% in organic revenue growth.
Perhaps one of the main advantages of the data lake, especially for organizations interested in getting ahead of the competition,
The Downside
Here’s the but… despite all these benefits, businesses continue to struggle with certain aspects of data delivery and integration. In fact, research shows that data scientists can spend up to 80 per cent of their time on these tasks – not the most efficient way of working!
So why are they struggling? First, unfortunately, storing data in its original form does not remove the need to adapt it later for machine learning processes, and this can become really complex. Over the last few years, data preparation tools have emerged specifically to try and make simple integration tasks more accessible to data scientists. These tools
Furthermore, having all your data in the same physical place doesn’t exactly make the discovery part easy. Think about it, it’s like the modern-day, digital equivalent of finding a needle in a haystack. In addition, big companies today have hundreds of repositories distributed on-premise platforms, data
So, What’s the Solution?
Ultimately, these issues with delivery and integration need to be addressed for organizations to unlock the full benefits of the data lake. Step forward, data virtualization.
Regardless of where your data is located or the format it is in, data virtualization provides a single access point by stitching together data abstracted from various underlying sources and delivering it to the consuming applications in real time. This way, even data that has still not been copied to the lake is available for data scientists.
In addition, it also helps to address other challenges faced by data scientists:
- Data discovery: Data virtualization provides a single point to expose all available data to
the consumers . Data virtualization is user-friendly, especially those tools with datacataloging capabilities which allow data scientists to search and browse all the data sets available. The technology liberates users and organizations alike by democratizing the data and providing a fast,cost effective way to access it - Data integration: The data is organized according to a consistent data representation and query model, meaning regardless of where the data is originally stored, data scientists can view all their data as if it were stored in the same place. It’s possible to make reusable logical data sets which can be adapted to meet the needs of each individual machine learning process, taking the pain out of data integration and preparation for data scientists.
Improving the Productivity of Data Scientists
The machine learning market is expected to grow by 44 per cent over the next four years, as companies seek ever more meaningful insight. As businesses continue to look to modern analytics and machine learning as a means of improving their operational efficiency, the need for technologies like data virtualization will also grow.
By enabling data scientists to discover and integrate data with ease, data virtualization can support them in exposing the results of machine learning
Useful Resources
- [Webinar on-demand] Minimizing the Complexities of Machine Learning with Data Virtualization
- [Webinar on-demand] Advanced Analytics and Machine Learning with Data Virtualization
- [Case study] Data Virtualization Seasons the Machine Learning and Blockchain Landscape for McCormick
- [Article] Data virtualisation: the key to better machine learning?
- Performance in Logical Architectures and Data Virtualization with the Denodo Platform and Presto MPP - September 28, 2023
- Beware of “Straw Man” Stories: Clearing up Misconceptions about Data Virtualization - November 11, 2021
- Why Data Mesh Needs Data Virtualization - August 19, 2021